‘What’s the worst thing that could happen?’ Some people use this phrase to motivate them to do something difficult, like give a speech or enter a social situation where they do not know anyone. It’s essentially the same as saying, “You’ve got nothing to lose.”
But for those who work in enterprise risk management, it’s a valuable question for just the opposite reason – an organization that makes a major misstep in managing risk has everything to lose. Knowing the “worst thing” that could happen, and the potential consequences, is critical knowledge to help lead key strategic decision-making.
It’s especially important in a fiercely competitive global business landscape where companies face multifaceted challenges that threaten success. For visionary enterprises, the application of data science is a pivotal tool, providing analytical capabilities to assess and monitor risk factors and confront intricate challenges head-on.
What Is Enterprise Risk Management?
Enterprise Risk Management (ERM) is a strategic approach that organizations use to identify and mitigate risks that could impede reaching goals and prevent success. For every industry, the risks are different. But the goal of ERM remains the same in every situation: provide a framework that considers the interconnected nature of risks and their potential impact on business operations.
The first step in ERM involves identification of risks that could impact the achievement of strategic goals. Risks manifest in various forms, including financial, operational, strategic, compliance-related, and reputational risks. Once identified, risks are assessed to determine their likelihood of occurrence and the potential impact they could have on the organization.
ERM involves developing strategies to manage and mitigate identified risks. These strategies may include risk avoidance, risk reduction, risk transfer (such as through insurance) or acceptance of certain risks when the potential benefits outweigh the drawbacks. Many companies also integrate ERM with strategic planning, allowing decision-makers to have more information when balancing risk vs. reward.
Data Science’s Crucial Role in ERM
Crafting an ERM plan is a complex endeavor. The success of enterprise risk management plans hinges on extracting insights from large datasets. Adding to the complications are the variety of risks that organizations can face. They include:
- Physical risk: A fire in an office building or warehouse.
- Location risks: Areas where natural disasters such as hurricanes or forest fires are more likely.
- Human risks: Risks caused by employee actions or theft.
- Financial risks: The risk of putting a great deal of cash into developing a new product, opening a new business location, or entering a new market.
- Technology risks: Computer system shutdowns or sudden loss of power.
Risks are generally ranked by their probability of occurring. Plans then are developed to mitigate those risks by preparing for the most likely events. Data analytics plays a critical role in making more accurate risk assessments for organizations facing various potential risks.
EY published a report that looked at the role of data in risk assessment and mitigation. It called for a shift to what EY called “risk enabled performance management,” a data-driven approach that focuses on breaking down potential risks to business drivers at a granulator level. Using data, the report stated, also eliminates subjectivity and individual perceptions.
The report also stated: “Today, data availability is limitless, technology is getting smarter and sharper, and business dynamics are more challenging than ever before. With this constantly increasing complexity and speed of change, harnessing the power of an effective risk management process has become imperative for the strategic and long-term success of an organization.”
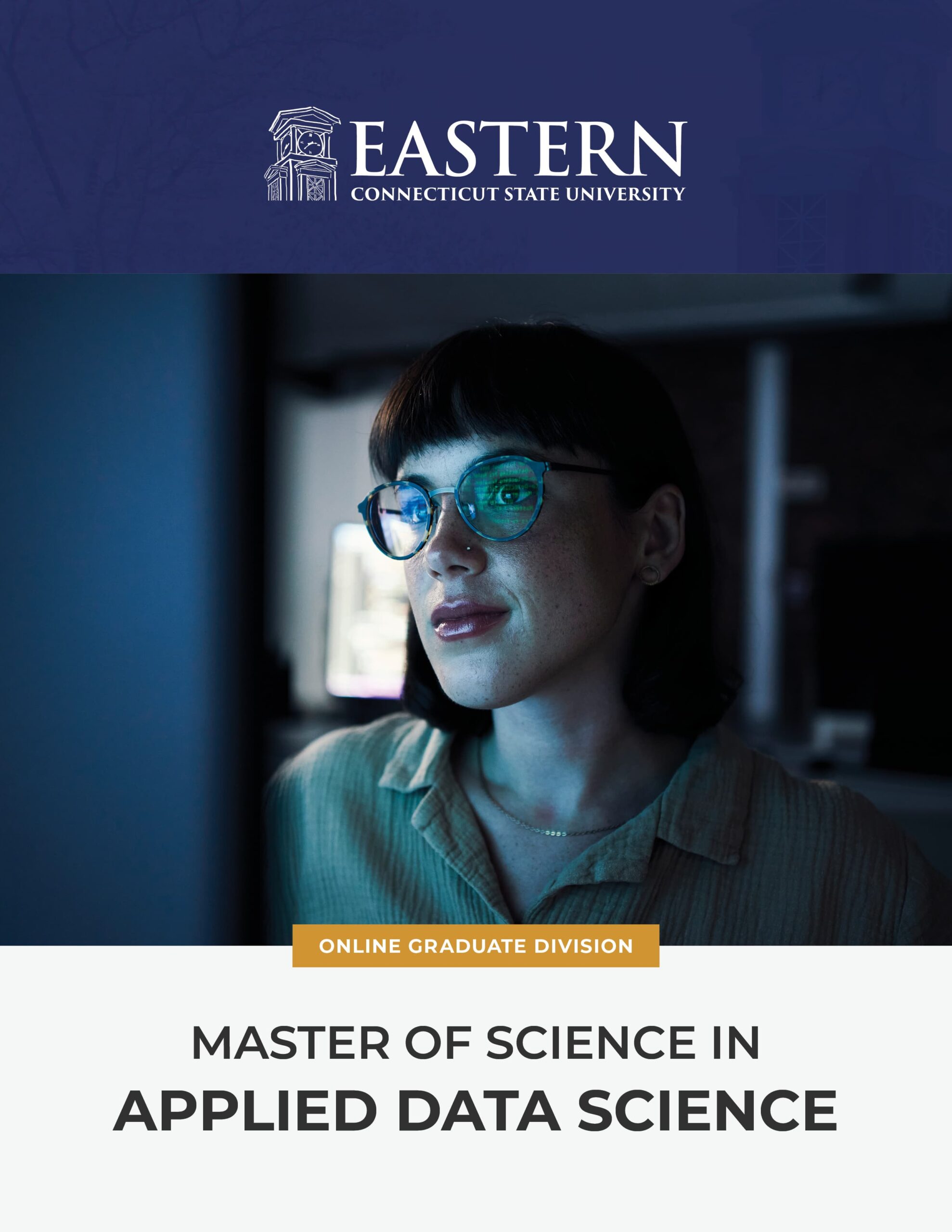
Earn an M.S. in Applied Data Science
Dive into the world of analytics with an online M.S. in Applied Data Science. Unlock actionable insights, master data-driven decision-making, and open doors to new opportunities. Begin your transformative journey now.
The Surge in Demand for Data Science Expertise
At the forefront of ERM, data scientists find themselves in high demand. The U.S. Bureau of Labor Statistics (BLS) projects a substantial 23% increase in computer and information research scientists over the next decade. Additionally, the BLS projects a 35% job growth for data scientists through 2032.
Between new job openings and the need to replace data scientists who retire from the field, the BLS projects approximately 17,000 job openings for data scientists every year, on average, over the next decade. The profession’s salary potential has also risen with demand. For example, data scientists earn a median annual salary of $103,500 nationally, according to the BLS, with those in the scientific research and development services industry earning $118,620 on average, while others in the management of companies and enterprises earn $106,510.
Explore Data Science Education
Enterprise risk management is a critical aspect of navigating the complexities of a competitive global business environment. Those who earn a Master of Science in Applied Data Science from Eastern Connecticut State University possess the skills and expertise in analytics that help organizational leaders make informed, data-driven strategic decisions and position themselves to play a crucial role in ensuring strategic and long-term success in risk management.
National long-term projections may not reflect local and/or short-term economic or job conditions, and do not guarantee actual job growth. The information provided is not intended to represent a complete list of hiring companies or job titles, and program options do not guarantee career or salary outcomes. Students should conduct independent research for specific employment information.